Concise Course in Advanced Level Statistics With Worked Examples Fourth Edition by Joan Sybil Chambers, Crawshaw, Brian Jefferson, David Bowles, Eddie Mullan, Garry Wiseman, John – Ebook PDF Instant Download/Delivery: 1382018010, 9781382018012
Full dowload Concise Course in Advanced Level Statistics With Worked Examples Fourth Edition after payment
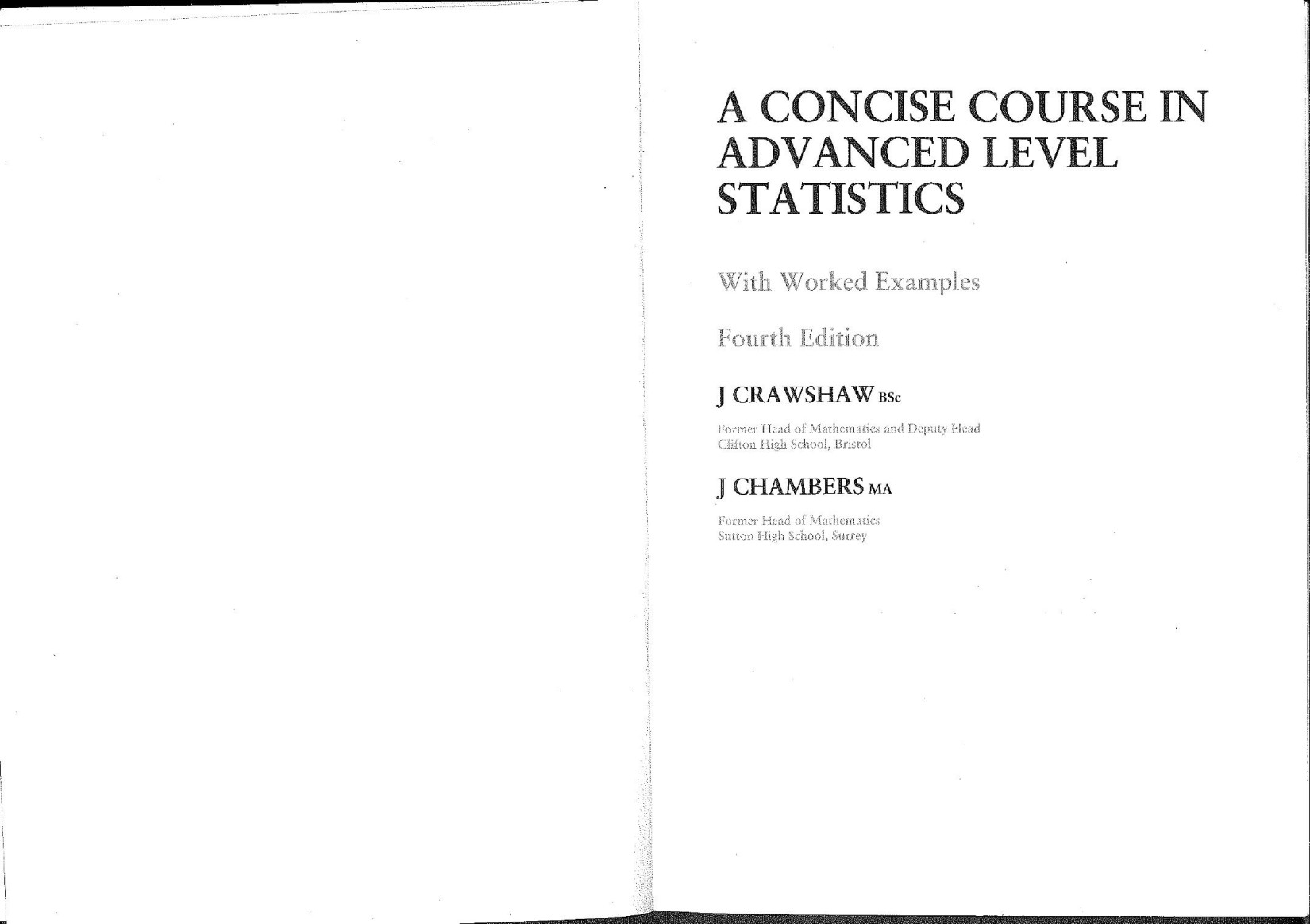
Product details:
ISBN 10: 1382018010
ISBN 13: 9781382018012
Author: Joan Sybil Chambers; D J Crawshaw; Brian Jefferson; David Bowles; Eddie Mullan; Garry Wiseman; John
This best-selling book remains the most popular stand-alone text for Advanced Level Statistics. It covers the AS and A2 specifications in Statistics for Advanced Level Maths across all boards.
Concise Course in Advanced Level Statistics With Worked Examples Fourth Table of contents:
1 Representation and summary of data
Discrete data
Continuous data
Stem and leaf diagrams (stemplots)
Ways of grouping data
Histograms
Frequency polygons
Frequency curves
Circular diagrams or pie charts
The mean
Variability of data
The standard deviation, s, and the variance, s²
Combining sets of data
Scaling sets of data
Using a method of coding to find the mean and standard deviation
Cumulative frequency
Cumulative percentage frequency diagrams
Median, quartiles and percentiles
Skewness
The normal distribution
Box and whisker diagrams (box plots)
Summary
2 Regression and correlation
Scatter diagrams
Regression function
Linear correlation and regression lines
The product-moment correlation coefficient, r
Spearman’s coefficient of rank correlation, r
Summary
3 Probability
Experimental probability
Probability when outcomes are equally likely
Subjective probabilities
Probability notation and probability laws
Illustrating two or more events using Venn diagrams
Probability rule for combined events
Exclusive (or mutually exclusive) events
Exhaustive events
Conditional probability
Independent events
Probability trees
Bayes’ Theorem
Some useful methods
Arrangements
Permutations of r objects from n objects
Combinations of r objects from n objects
Summary
4 Probability distributions I – discrete variables
Probability distributions
Expectation of X, E(X)
Expectation of any function of X, E(g(X))
Variance, Var(X) or V(X)
The Cumulative distribution function, F(x)
Two independent random variables
Distribution of X₁ + X₂ +⋯+ X
Comparing the distributions of X₁ + X₂ and 2X
Summary
5 Special discrete probability distributions
The uniform distribution
The geometric distribution
Expectation and variance of the geometric distribution
The binomial distribution
Expectation and variance of the binomial distribution
The Poisson distribution
Using the Poisson distribution as an approximation to the binomial distribution
The sum of independent Poisson variables
Summary
6 Probability distributions II – continuous variables
Continuous random variables
Probability density function (p.d.f.)
Expectation of X, E(X)
Expectation of any function of X
Variance of X, Var(X)
The mode
Cumulative distribution function F(x)
Obtaining the p.d.f., f(x), from the cumulative distribution function
The continous uniform (or rectangular) distribution
Expectation and variance of the uniform distribution
The cumulative distribution function, F(x), for a uniform distribution
Summary
7 The normal distribution
Finding probabilities
The standard normal variable, Z
Using standard normal tables
Using standard normal tables for any normal variable, X
Using the standard normal tables in reverse to find z when Φ(z) is known
Using the tables in reverse for any normal variable, X
Value of μ or σ or both
The normal approximation to the binomial distribution
Continuity corrections
Deciding when to use a normal approximation and when to use a Poisson approximation for a binomial d
The normal approximation to the Poisson distribution
Summary
8 Linear combinations of normal variables
The sum of independent normal variables
The difference of independent normal variables
Multiples of independent normal variables
Summary
9 Sampling and estimation
Sampling
Surveys
Sampling methods
Simulating random samples from given distributions
Sample statistics
The distribution of the sample mean
Central limit theorem
The distribution of the sample proportion, p
Unbiased estimates of population parameters
Point estimates
Interval estimates
The t-distribution
Confidence intervals for the population proportion, p
Summary
10 Hypothesis tests: discrete distributions
Hypothesis test for a binomial proportion, p (small sample size)
Procedure for carrying out a hypothesis test
One-tailed and two-tailed tests
Summary of stages of a hypothesis (significance) test
Type I and Type II errors
Significance test for a Poisson mean λ
Summary of stages of a significance test
Summary of Type I and Type II errors
11 Hypothesis testing (z-tests and t-tests)
Hypothesis testing
One-tailed and two-tailed tests
Critical z-values
Summary of critical values and rejection criteria
Stages in the hypothesis test
Hypothesis test 1: testing μ (the mean of a population)
Type I and Type II errors
Hypothesis test 2: testing a binomial proportion p when n is large
Hypothesis test 3: testing μ₁ − μ₂, the difference between means of two normal populations
Summary
12 The χ² significance test
The χ² significance test
Performing a χ² goodness-of-fit test
Summary of the procedure for performing a χ² goodness-of-fit test
Test 1 – goodness-of-fit test for a uniform distribution
Test 2 – goodness-of-fit test for a distribution in a given ratio
Test 3 – goodness-of-fit test for a binomial distribution
Test 4 – goodness-of-fit test for a Poisson distribution
Test 5 – goodness-of-fit test for a normal distribution
Summary of the number of degrees of freedom for a goodness-of-fit test
The χ² significance test for independence
Summary
13 Significance tests for correlation coefficients
Significance tests for correlation coefficients
Test for the product-moment correlation coefficient, r
Spearman’s coefficient of rank correlation, r
Summary
ICT statistics supplement