Molecular Evolution 1st editon by Ziheng Yang – Ebook PDF Instant Download/Delivery: 0199602603, 9780199602605
Full dowload Molecular Evolution 1st editon after payment
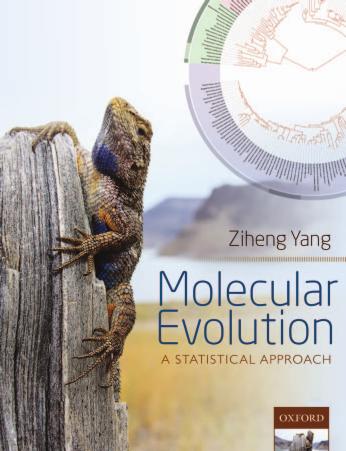
Product details:
ISBN 10: 0199602603
ISBN 13: 9780199602605
Author: Ziheng Yang
Molecular Evolution 1st Table of contents:
1. Models of nucleotide substitution
1.1. Introduction
1.2. Markov models of nucleotide substitution and distance estimation
1.2.1. The JC69 model
1.2.2. The K80 model
1.2.3. HKY85, F84, TN93, etc.
1.2.4. The transition/transversion rate ratio
1.3. Variable substitution rates across sites
1.4. Maximum likelihood estimation of distance
1.4.1. The JC69 model
1.4.2. The K80 model
1.4.3. Likelihood ratio test of substitution models
*1.4.4. Profile and integrated likelihood methods
1.5. Markov chains and distance estimation under general models
1.5.1. Markov chains
*1.5.2. Distance under the unrestricted (UNREST) model
*1.5.3. Distance under the general time-reversible model
1.6. Discussions
1.6.1. Distance estimation under different substitution models
1.6.2. Limitations of pairwise comparison
1.7. Problems
2. Models of amino acid and codon substitution
2.1. Introduction
2.2. Models of amino acid replacement
2.2.1. Empirical models
2.2.2. Mechanistic models
2.2.3. Among-site heterogeneity
2.3. Estimation of distance between two protein sequences
2.3.1. The Poisson model
2.3.2. Empirical models
2.3.3. Gamma distances
2.4. Models of codon substitution
2.4.1. The basic model
2.4.2. Variations and extensions
2.5. Estimation of dS and dN
2.5.1. Counting methods
2.5.2. Maximum likelihood method
2.5.3. Comparison of methods
2.5.4. More distances and interpretation of the dN/dS ratio
2.5.5. Estimation of dS and dN in comparative genomics
*2.5.6. Distances based on the physical-site definition
*2.5.7. Utility of the distance measures
*2.6. Numerical calculation of the transition probability matrix
2.7. Problems
3. Phylogeny reconstruction: overview
3.1. Tree concepts
3.1.1. Terminology
3.1.2. Species trees and gene trees
3.1.3. Classification of tree reconstruction methods
3.2. Exhaustive and heuristic tree search
3.2.1. Exhaustive tree search
3.2.2. Heuristic tree search
3.2.3. Branch swapping
3.2.4. Local peaks in the tree space
3.2.5. Stochastic tree search
3.3. Distance matrix methods
3.3.1. Least-squares method
3.3.2. Minimum evolution method
3.3.3. Neighbour-joining method
3.4. Maximum parsimony
3.4.1. Brief history
3.4.2. Counting the minimum number of changes on a tree
3.4.3. Weighted parsimony and dynamic programming
3.4.4. Probabilities of ancestral states
3.4.5. Long-branch attraction
3.4.6. Assumptions of parsimony
3.5. Problems
4. Maximum likelihood methods
4.1. Introduction
4.2. Likelihood calculation on tree
4.2.1. Data, model, tree, and likelihood
4.2.2. The pruning algorithm
4.2.3. Time reversibility, the root of the tree, and the molecular clock
4.2.4. A numerical example: phylogeny of apes
4.2.5. Amino acid, codon, and RNA models
*4.2.6. Missing data, sequence errors, and alignment gaps
4.3. Likelihood calculation under more complex models
4.3.1. Mixture models for variable rates among sites
4.3.2. Mixture models for pattern heterogeneity among sites
4.3.3. Partition models for combined analysis of multiple datasets
4.3.4. Nonhomogeneous and nonstationary models
4.4. Reconstruction of ancestral states
4.4.1. Overview
4.4.2. Empirical and hierarchical Bayesian reconstruction
*4.4.3. Discrete morphological characters
4.4.4. Systematic biases in ancestral reconstruction
*4.5. Numerical algorithms for maximum likelihood estimation
*4.5.1. Univariate optimization
*4.5.2. Multivariate optimization
4.6. ML optimization in phylogenetics
4.6.1. Optimization on a fixed tree
4.6.2. Multiple local peaks on the likelihood surface for a fixed tree
4.6.3. Search in the tree space
4.6.4. Approximate likelihood method
4.7. Model selection and robustness
4.7.1. Likelihood ratio test applied to rbcL dataset
4.7.2. Test of goodness of fit and parametric bootstrap
*4.7.3. Diagnostic tests to detect model violations
4.7.4. Akaike information criterion (AIC and AICc)
4.7.5. Bayesian information criterion
4.7.6. Model adequacy and robustness
4.8. Problems
5. Comparison of phylogenetic methods and tests on trees
5.1. Statistical performance of tree reconstruction methods
5.1.1. Criteria
5.1.2. Performance
5.2. Likelihood
5.2.1. Contrast with conventional parameter estimation
5.2.2. Consistency
5.2.3. Efficiency
5.2.4. Robustness
5.3. Parsimony
5.3.1. Equivalence with misbehaved likelihood models
5.3.2. Equivalence with well-behaved likelihood models
5.3.3. Assumptions and justifications
5.4. Testing hypotheses concerning trees
5.4.1. Bootstrap
5.4.2. Interior-branch test
5.4.3. K-H test and related tests
5.4.4. Example: phylogeny of apes
5.4.5. Indexes used in parsimony analysis
5.5. Problems
6. Bayesian theory
6.1. Overview
6.2. The Bayesian paradigm
6.2.1. The Bayes theorem
6.2.2. The Bayes theorem in Bayesian statistics
*6.2.3. Classical versus Bayesian statistics
6.3. Prior
6.3.1. Methods of prior specification
6.3.2. Conjugate priors
6.3.3. Flat or uniform priors
*6.3.4. The Jeffreys priors
*6.3.5. The reference priors
6.4. Methods of integration
*6.4.1. Laplace approximation
6.4.2. Mid-point and trapezoid methods
6.4.3. Gaussian quadrature
6.4.4. Marginal likelihood calculation for JC69 distance estimation
6.4.5. Monte Carlo integration
6.4.6. Importance sampling
6.5. Problems
7. Bayesian computation (MCMC)
7.1. Markov chain Monte Carlo
7.1.1. Metropolis algorithm
7.1.2. Asymmetrical moves and proposal ratio
7.1.3. The transition kernel
7.1.4. Single-component Metropolis–Hastings algorithm
7.1.5. Gibbs sampler
7.2. Simple moves and their proposal ratios
7.2.1. Sliding window using the uniform proposal
7.2.2. Sliding window using the normal proposal
7.2.3. Bactrian proposal
7.2.4. Sliding window using the multivariate normal proposal
7.2.5. Proportional scaling
7.2.6. Proportional scaling with bounds
7.3. Convergence, mixing, and summary of MCMC
7.3.1. Convergence and tail behaviour
7.3.2. Mixing efficiency, jump probability, and step length
7.3.3. Validating and diagnosing MCMC algorithms
7.3.4. Potential scale reduction statistic
7.3.5. Summary of MCMC output
7.4. Advanced Monte Carlo methods
7.4.1. Parallel tempering (MC3)
7.4.2. Trans-model and trans-dimensional MCMC
7.4.3. Bayes factor and marginal likelihood
7.5. Problems
8. Bayesian phylogenetics
8.1. Overview
8.1.1. Historical background
8.1.2. A sketch MCMC algorithm
8.1.3. The statistical nature of phylogeny estimation
8.2. Models and priors in Bayesian phylogenetics
8.2.1. Priors on branch lengths
8.2.2. Priors on parameters in substitution models
8.2.3. Priors on tree topology
8.3. MCMC proposals in Bayesian phylogenetics
8.3.1. Within-tree moves
8.3.2. Cross-tree moves
8.3.3. NNI for unrooted trees
8.3.4. SPR for unrooted trees
8.3.5. TBR for unrooted trees
8.3.6. Subtree swapping
8.3.7. NNI for rooted trees
8.3.8. SPR on rooted trees
8.3.9. Node slider
8.4. Summarizing MCMC output
8.5. High posterior probabilities for trees
8.5.1. High posterior probabilities for trees or splits
8.5.2. Star tree paradox
*8.5.3. Fair coin paradox, fair balance paradox, and Bayesian model selection
8.5.4. Conservative Bayesian phylogenetics
8.6. Problems
9. Coalescent theory and species trees
9.1. Overview
9.2. The coalescent model for a single species
9.2.1. The backward time machine
9.2.2. Fisher–Wright model and the neutral coalescent
9.2.3. A sample of n genes
9.2.4. Simulating the coalescent
9.2.5. Estimation of θ from a sample of DNA sequences
9.3. Population demographic process
9.3.1. Homogeneous and nonhomogeneous Poisson processes
9.3.2. Deterministic population size change
9.3.3. Nonparametric population demographic models
9.4. Multispecies coalescent, species trees and gene trees
9.4.1. Multispecies coalescent
9.4.2. Species tree–gene tree conflict
9.4.3. Estimation of species trees
9.4.4. Migration
9.5. Species delimitation
9.5.1. Species concept and species delimitation
9.5.2. Simple methods for analysing genetic data
9.5.3. Bayesian species delimitation
9.5.4. The impact of guide tree, prior, and migration
9.5.5. Pros and cons of Bayesian species delimitation
9.6. Problems
10. Molecular clock and estimation of species divergence times
10.1. Overview
10.2. Tests of the molecular clock
10.2.1. Relative-rate tests
10.2.2. Likelihood ratio test
10.2.3. Limitations of molecular clock tests
10.2.4. Index of dispersion
10.3. Likelihood estimation of divergence times
10.3.1. Global clock model
10.3.2. Local clock model
10.3.3. Heuristic rate-smoothing methods
10.3.4. Uncertainties in calibrations
10.3.5. Dating viral divergences
10.3.6. Dating primate divergences
10.4. Bayesian estimation of divergence times
10.4.1. General framework
10.4.2. Approximate calculation of likelihood
10.4.3. Prior on evolutionary rates
10.4.4. Prior on divergence times and fossil calibrations
10.4.5. Uncertainties in time estimates
10.4.6. Dating viral divergences
10.4.7. Application to primate and mammalian divergences
10.5. Perspectives
10.6. Problems
11. Neutral and adaptive protein evolution
11.1. Introduction
11.2. The neutral theory and tests of neutrality
11.2.1. The neutral and nearly neutral theories
11.2.2. Tajima’s D statistic
11.2.3. Fu and Li’s D, and Fay and Wu’s H statistics
11.2.4. McDonald–Kreitman test and estimation of selective strength
11.2.5. Hudson–Kreitman–Aquade test
11.3. Lineages undergoing adaptive evolution
11.3.1. Heuristic methods
11.3.2. Likelihood method
11.4. Amino acid sites undergoing adaptive evolution
11.4.1. Three strategies
11.4.2. Likelihood ratio test of positive selection under random-site models
11.4.3. Identification of sites under positive selection
11.4.4. Positive selection at the human MHC
11.5. Adaptive evolution affecting particular sites and lineages
11.5.1. Branch-site test of positive selection
11.5.2. Other similar models
11.5.3. Adaptive evolution in angiosperm phytochromes
11.6. Assumptions, limitations, and comparisons
11.6.1. Assumptions and limitations of current methods
11.6.2. Comparison of methods for detecting positive selection
11.7. Adaptively evolving genes
11.8. Problems
12. Simulating molecular evolution
12.1. Introduction
12.2. Random number generator
12.3. Generation of discrete random variables
12.3.1. Inversion method for sampling from a general discrete distribution
12.3.2. The alias method for sampling from a discrete distribution
12.3.3. Discrete uniform distribution
12.3.4. Binomial distribution
12.3.5. The multinomial distribution
12.3.6. The Poisson distribution
12.3.7. The composition method for mixture distributions
12.4. Generation of continuous random variables
12.4.1. The inversion method
12.4.2. The transformation method
12.4.3. The rejection method
12.4.4. Generation of a standard normal variate using the polar method
12.4.5. Gamma, beta, and Dirichlet variables
12.5. Simulation of Markov processes
12.5.1. Simulation of the Poisson process
12.5.2. Simulation of the nonhomogeneous Poisson process
12.5.3. Simulation of discrete-time Markov chains
12.5.4. Simulation of continuous-time Markov chains
12.6. Simulating molecular evolution
12.6.1. Simulation of sequences on a fixed tree
12.6.2. Simulation of random trees
12.7. Validation of the simulation program
12.8. Problems
People also search for Molecular Evolution 1st:
molecular evolution quizlet
evolution molecular biology
evolution molecular evidence
molecular biology evolution examples